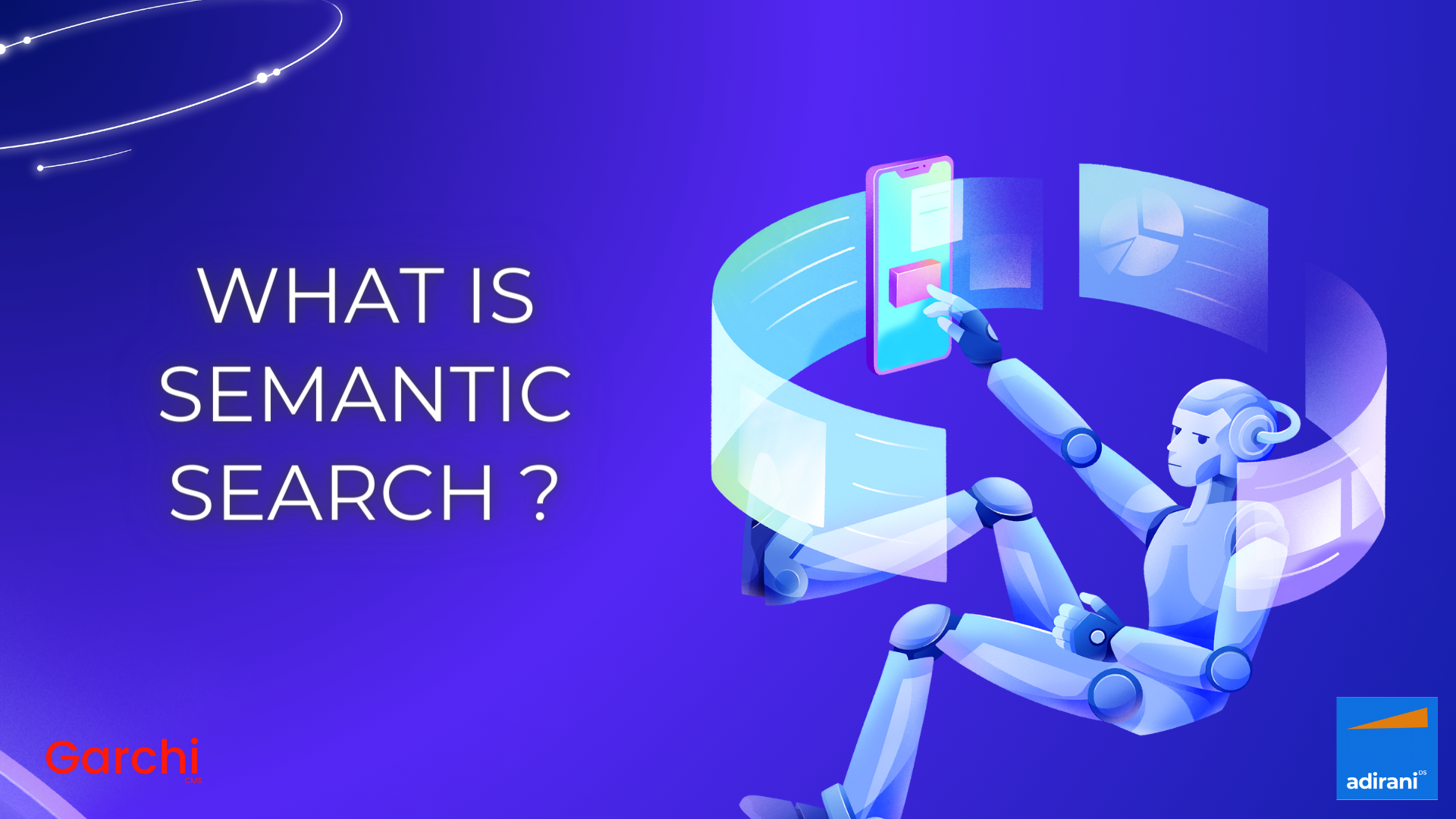
Semantic Search
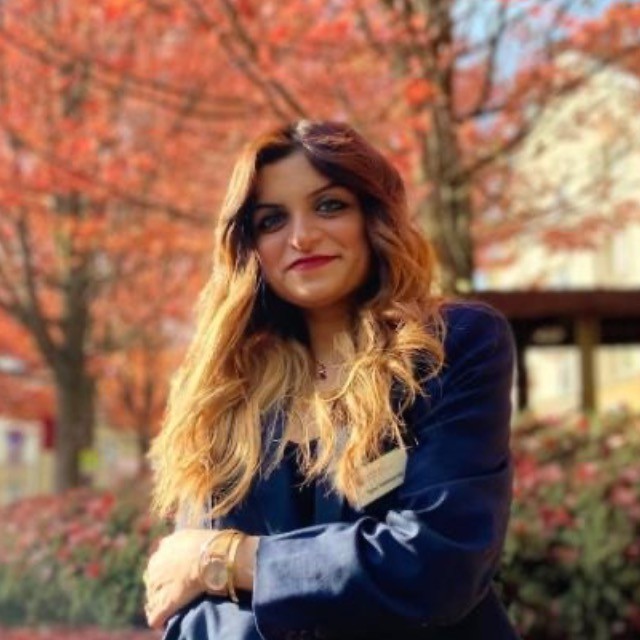
Imagine typing 'best coffee places near me' into a search engine and, instead of getting a random list of coffee shops, you're presented with cosy cafes that match your love for organic blends and indie music. This is a semantic search at work. Semantic search goes beyond the surface of words to grasp the intent and context of your queries, offering results that feel surprisingly personal. Now, isn't that a refreshing way to start your search – and your day?
What is Semantic Search?
I'm someone who prefers to understand the query logic than just the surface of typing out a search query; I'm fascinated by the 'why' and 'how' behind it. That's precisely where Semantic Search plays its part. It’s not limited to the 'what' of your query. Instead, it digs into the reasons and the methods behind it. Through sophisticated algorithms, it picks up on the subtle nuances of our language - be it idioms, synonyms, or even the specific quirks of regional dialects. This approach goes beyond the realm of traditional keyword matching. It leverages the power of Natural Language Processing (NLP) and Machine Learning (ML) to truly understand not just the words we type but the intent and meaning we embed within them.
Imagine engaging in a dialogue with someone who is part linguist, part psychologist - the search engine becomes an entity that comprehends your language and intentions. This is the kind of intelligent, user-centric approach that elevates our search experiences to new heights, making them not only more efficient but also more intuitive and personalized.
Why is Semantic Search a Game Changer for User Engagement?
Imagine a user searching for lifestyle products on your platform. With Semantic Search, their journey becomes more than a transaction; it becomes a personalized experience. Whether they are casually browsing or looking for something specific, the technology ensures that they find exactly what they need, and often, something more. This isn't just about enhancing user satisfaction; it's about transforming their interaction into a series of meaningful discoveries. The impact? Your customers spend less time searching and more time engaging with content that resonates with them, leading to higher conversion rates and, importantly, a stronger connection with your brand.
Google is perhaps the most prominent example of a search engine that utilizes semantic search extensively. Over the years, Google has continuously refined its algorithms to better understand the intent behind users' queries. With updates like Hummingbird and the introduction of the Knowledge Graph, Google has shifted from mere keyword matching to understanding the context and relationships between words and phrases in a query. This allows Google to provide more accurate and contextually relevant search results.
You can see the knowledge graph in action on the results page yielded upon searching for “chocolate chip cookies.” The SERP does contain standard organic results and links to suitable websites, but it also contains a rich set of knowledge graph data, including an answer box with a recipe, a right-hand knowledge panel featuring nutritional facts about this dessert, and suggestions for related search subjects.
To understand the process of Semantic Search, it's helpful to look at the steps involved clearly and concisely. Initially, the journey starts with data collection, often organized in a CSV file. This data is the backbone of the search system. The next step is to feed this data into an embedding model. In this stage, the model converts the data into a series of vectors. These vectors are crucial because they represent the data in a format that the search system can efficiently process.
Once we have these vectors, they are stored in a system ready for retrieval. When a user enters a query, the system begins its work. It first encodes the user's query into a similar vector format. With both the query and the data now in vector form, the system uses a method called ‘cosine similarity’ to compare them. This method calculates how closely the query vector matches the data vectors.
The closer the match, the more relevant the result. This process allows the search engine to retrieve and present results that are not just based on keywords but are aligned with the user's intent and the context of their query. It's a methodical process, combines data management, machine learning, and sophisticated matching techniques to deliver a search experience that is both intuitive and precise.
In Semantic Search, the cosine similarity rule is used to determine how similar two pieces of information are. The formula for this is quite elegant in its simplicity. It calculates the cosine of the angle between two vectors – think of these vectors as arrows pointing in different directions. The formula looks like this:
Cosine Similarity Rule –
Here, A⋅B represents the dot product of the two vectors, which is a way of multiplying them. The bottom part, ∥A∥∥B∥, is the magnitude (or length) of each vector. When the vectors are very similar, the angle between them is small, and the cosine similarity is close to 1. If they're very different, the angle is larger, and the cosine similarity gets closer to 0. This formula helps the search engine to understand how closely the content of your query matches potential search results, ensuring that the results you see are relevant to what you're looking for.
Process of Semantic Search
Example in action:
Let's see an example of a semantic search module in action.
We will first need a data set/resource to perform a semantic search on. For this, I will download all the blog article data that I have on Garchi CMS
The downloaded CSV files have 15 columns and around 20 articles. We can run the embedding model of Open AI against the article body which is a rich text-long description under the column named "Detailed Description". The problem here is that the content is in HTML format so we need to convert it to plain text by removing the html tags. When it comes to embedding the text, the bigger the text better the embedding results. The good thing is as it has quite a good amount of text (around 800-1000 words for each article) it is perfect for embedding.
Open AI Embedding API has a token limit for the text embedding so we need to make sure to chunk the bigger text and then take the average of the embeddings of these chunks of text. This is just one of the methods but feel free to use your method to solve this problem.
So the steps for creating our semantic search model would be as below
- Data cleaning (removing HTML tags) and chunking the text to embed it.
- Store the embeddings in a vector database or vector column (in the case of PGSQL). This step I will be skipping as the main intention is to demonstrate how a simple semantic search model works.
- Get the search query from the user and embed it
- Run the cosine similarity function for each embedded description and embedded search query. Put the threshold to pick the most accurate match.
Now let's translate these steps to Python code. We will need to install some packages to make our lives easy 😆
pip install pandas numpy beautifulsoup4 openai
We will now create a class named OpenAIService in OpenAIService.py file. The purpose of this class is to have the functions required to create embeddings and run cosine similarity.
import openai
from openai.embeddings_utils import cosine_similarity
class OpenAIService:
def __init__(self):
openai.api_key = "your_openai_api_key"
# uses openai embedding api to create vector embedding of the text supplied as input string or list of strings
def create_embeddings(self, input: str | list[str]):
response = openai.Embedding.create(
model="text-embedding-ada-002",
input=input
)
return response['data']
# uses cosine_similarity function from openai utilities to get cosine similarity which is always between 0-1
def getSimilarities(self, embedding1: list[float], embedding2: list[float]):
return cosine_similarity(embedding1, embedding2)
We will now create a SemanticSearch class in the SemanticSearch.py file.
import pandas as pd
from OpenAIService import OpenAIService
from bs4 import BeautifulSoup
import numpy as np
class SemanticSearch:
def __init__(self):
self.df = pd.read_csv("items.csv")
self.openaiservice = OpenAIService()
def cleanHTML(self):
self.df["Detailed Description"] = self.df["Detailed Description"].apply(lambda x: BeautifulSoup(x, "html.parser").text)
def split_text(self, text, max_length=8000):
# Open AI model has character limit of 8192 tokens. So we splits the text into chunks of max_length characters
return [text[i:i+max_length] for i in range(0, len(text), max_length)]
def create_embeddings_for_text(self, text):
# Split the text into chunks
chunks = self.split_text(text)
# Create embeddings for each chunk and then combine
embeddings = [self.openaiservice.create_embeddings(chunk)[0]['embedding'] for chunk in chunks]
# Average the embeddings (or choose another method to combine them)
avg_embedding = np.mean([np.array(embedding) for embedding in embeddings], axis=0)
return avg_embedding.tolist()
def search(self, query: str):
self.cleanHTML()
self.df["embedding"] = self.df["Detailed Description"].apply(self.create_embeddings_for_text)
query_embedding = self.create_embeddings_for_text(query)
self.df["similarity"] = self.df["embedding"].apply(lambda emb: self.openaiservice.getSimilarities(query_embedding, emb))
# put a threshold for similarity
self.df = self.df[self.df["similarity"] > 0.80]
self.df.sort_values(by="similarity", ascending=False, inplace=True)
return self.df['Item Name'].head(5).tolist()
smSearch = SemanticSearch()
print(smSearch.search("SQL articles"))
Let's try to break down this code from SemanticSearch.py
The smSearch
is the object of class SemanticSearch which we will be using to run the search function. The function needs the user's search query which we have hardcoded for now to "SQL Articles". The search() calls the clean_html() then create_embeddings_for_text() and finally calculates cosine similarities.
The constructor of this class will load the CSV file that we downloaded from Garchi CMS and then initialise the OpenAIService class object to use the functions from OpenAIService class. The cleanHTML() will remove all the HTML tags and make the detailed description (body) of each blog article into plain text.
We will then apply create_embeddings_for_text() to each detailed description of the article. The function splits the text into a chunk of max 8000 characters using the split_text function and then embeds the chunked text using openai API. Finally the function returns the average of chunked embeddings as a final embedding of entire text chunk.
As the cosine similarity runs from 0-1, we want the near accurate matches so I have put the threshold of 0.8 that means only those results' descriptions will be considered which are atleast 80% semantically match to the search_query asked by the user. We then arrange the result dataset in decending order of cosine_similarity and return max 5 results's Item Name which is the blog titles.
After we run SemanticSearch.py file here is the final result. You can find all these articles here
['Paginating data in Fast API', 'Discovering SQL: A Game Changer in My Data Journey', 'Website Builders vs Headless CMS: Where Does Garchi Fit In?', 'Compound Querying - Flexibility in Data Management']